Reversal Learning Task Eeg Study(Karcher Et Al 2017 Review
Abstract
Both positive psychotic symptoms and anhedonia are associated with striatal functioning, but few studies accept linked risk for psychotic disorders to a neural measure out evoked during a striatal dopamine-related reward and punishment-based learning task, such every bit a reversal learning chore (RLT; Cools et al, 2009). The feedback-related negativity (FRN) is a neural response that in part reflects striatal dopamine functioning. We recorded EEG during the RLT in iii groups: (a) people with psychotic experiences (PE; n=20) at increased risk for psychotic disorders; (b) people with extremely elevated social anhedonia (SocAnh; n=22); and (c) controls (n=twenty). Behaviorally, consistent with increased striatal dopamine, the PE grouping exhibited meliorate behavioral learning (ie, faster responses) after unexpected advantage than after unexpected punishment. Moreover, although the command and SocAnh groups showed a larger FRN to penalty than reward, the PE group showed similar FRNs to penalty and reward, with a numerically larger FRN to reward than penalty (with similar results on these trials also found for a P3a component). These results are among the kickoff to link a neural response evoked by a reward and punishment-based learning task specifically with elevated psychosis risk.
Principal
In psychotic disorders, there is evidence that the striatum might exist involved in both positive (eg, Howes and Kapur, 2009) and negative symptoms (eg, Juckel et al, 2006). Given confounds often present in patient enquiry (eg, medication furnishings; Demjaha et al, 2012), information technology is also important to examine associations betwixt disorder take a chance and striatal performance. However, very few studies have linked either psychotic experiences (ie, psychotic-like experiences that predict increased risk for psychotic disorder; Chapman et al, 1994; Kaymaz et al, 2012) or anhedonia in at-risk populations to a neural response evoked by a striatal dopamine-related behavioral task. Moreover, in at-run a risk populations, there is merely limited evidence of impairments in behavioral measures that are thought to exist related to striatal functioning. Arguably, the best existing show is that first-degree relatives of people with schizophrenia exhibit impairments on striatal-related behavioral measures (ie, on a probabilistic category learning task; eg, Wagshal et al, 2012; Weickert et al, 2010). Even so, information technology is non clear from this work whether these behavioral impairments are related to take a chance for positive psychotic symptoms or risk for negative symptoms (or neither). Here we examined whether either psychotic experiences (PE) or social anhedonia (SocAnh) in at-take chances groups is associated with behavioral and neural measures of reward and punishment-based learning.
A neural mensurate related to striatal dopamine is the feedback-related negativity (FRN), an event-related potential (ERP) component occurring ~250 ms following operation feedback appearing near robustly in frontocentral electrodes (eg, Carlson et al, 2011). The FRN is typically larger (ie, more negative) for unexpected negative than positive feedback (eg, Proudfit, 2015). Hence, the FRN is often measured as the difference between negative vs positive feedback (eg, Nieuwenhuis et al, 2005). The FRN has consistently been linked to activation in the striatum (eg, Carlson et al, 2011; Becker et al, 2014; although other regions are besides involved in its generation; eg, Baker and Holroyd, 2011).
In particular, the FRN is thought to be influenced by phasic changes in striatal dopamine (Becker et al, 2014; Walsh and Anderson, 2012). Consistent with this, administration of dopamine agonists has been found to increment the FRN specifically for right positive feedback trials (Santesso et al, 2009). Ane interpretation of this effect is that increased dopamine produces increased salience for positive feedback. This interpretation is consistent with bear witness that the FRN is influenced by the salience of outcomes (eg, Pfabigan et al, 2015), that increased striatal dopamine is associated with heightened sensitivity to rewards and decreased sensitivity to punishments (Collins and Frank, 2014), and that evidence of increased sensitivity to rewards is associated with increased FRN for unexpected rewards (Smillie et al, 2011). The reversal learning task (RLT) adult by Cools et al (2009) involves periodic, unexpected positive and negative feedback, with increased striatal dopamine (either baseline levels or due to pharmacological manipulation) associated with behavioral evidence of better learning subsequently unexpected reward relative to unexpected punishment (Cools et al, 2009; van der Schaaf et al, 2014). In the current report, behavioral performance on the RLT, measured meantime with the FRN, was used to index the extent to which abnormal reward and punishment-based learning is apparent in PE or SocAnh risk groups.
To our knowledge, no previous written report has examined the FRN or the RLT and risk for schizophrenia-spectrum disorders. We expected that the results for PE would exist consistent with abnormal reward and punishment-based learning (Karcher et al, 2015a). Behaviorally, we expected that PE would exist associated with better learning from unexpected reward than from unexpected penalisation and we also expected that PE would be associated with a relatively larger FRN for unexpected advantage than for unexpected punishment. As far equally SocAnh, based on possible deficits in processing rewards, we expected that SocAnh would be related to a relatively smaller FRN for unexpected reward than for unexpected punishment. Farther, to examine whether any associations with the FRN were not due to grouping differences in the P3a, a component that partially overlaps with the FRN's scalp distribution that is known to be highly sensitive to novelty (Polich, 2007) and that follows the FRN in time, we also examined P3a amplitudes on unexpected feedback trials (ie, to examine whether what appears to be a larger FRN could actually reflect a smaller P3a).
MATERIALS AND METHODS
Participants
The PE grouping consisted of twenty people (55% women; mean historic period=eighteen.45 years (SD=0.sixty); seventy% Caucasian) who scored >1.96 SD above the same-sex mean on the Perceptual Aberration (Chapman et al, 1978) or Magical Ideation (Eckblad and Chapman, 1983) scales or had a summed, standardized score from the Perceptual Aberration and Magical Ideation (PerMag) scales >3.0. People with extremely elevated PerMag scores take been found to exist at increased risk for psychotic disorders (Chapman et al, 1994). Further, all PE participants in the current written report besides had current interview ratings⩾ii (with two=presence of attenuated PE) on both Unusual Thought Content/Delusional Ideation and Perceptual Abnormalities/Hallucinations subscales of the Structured Interview for Prodromal Syndromes (SIPS; Miller et al, 2003; with the SIPS having been used successfully to predict chance for future psychotic disorder; current inter-rater reliabilities for two raters ⩾0.93; in the current written report, no participant scored a vi on whatever of the domains of the SIPS, indicating no frank psychosis).
The SocAnh group independent 22 people (65.ii% women; hateful age=xviii.74 years (SD=0.81); 65% Caucasian) who scored >one.96 SD higher up the same sexual practice hateful on the Revised SocAnh Scale (Eckblad et al, 1982). Extremely elevated SocAnh 'is rather selectively associated with the emergence of nonpsychotic schizophrenic-spectrum disorders' (Debbané et al, 2015). In order to more conspicuously distinguish psychosis take a chance from elevated SocAnh, to be recruited for this study the SocAnh (and control) groups had to be rated as never having PE (ie,<ii) on the SIPS.
There were 21 people in the control group (50% women; mean age=18.39 years (SD=0.78); 79% Caucasian) who scored <0.v SD below the mean on the Revised Social Anhedonia Calibration, Perceptual Aberration Calibration, and Magical Ideation Scale. All study participants were Introduction to Psychology students who participated for course credit after taking function in a separate behavioral testing session (Karcher et al, 2015b; see Supplementary Methods for recruitment details). In the current study, there were no significant differences between the three groups on any demographic variables (eg, sex, age, or ethnicity).
Materials
Reversal learning task
In general on reversal learning tasks, participants first learn that one stimulus is rewarded and that a 2nd stimulus is punished. Afterward, the associations with reward and penalty are switched and participants have to larn that the first stimulus is now punished and that the 2nd stimulus is now rewarded, with this pattern repeating through a number of reversals. There are two trial types that are near important for this task: (1) unexpected reward trials, where a stimulus that was previously associated with punishment is now associated with reward; and (ii) unexpected penalization trials, where a stimulus previously associated with advantage is now associated with penalty. On each trial two horizontally side by side stimuli were presented simultaneously, one face and i natural scene (eg, depicting a mountain range), 1 of which was highlighted with a yellow edge. The participant predicted whether the highlighted stimulus would lead to a reward or a punishment, indicating their prediction past pressing one of two buttons on a response box (both stimuli remained on-screen until the participant responded; Cools et al, 2009). The participant's self-paced response was followed immediately by an event message, presented for 500 ms (and following the outcome bulletin, there was an inter-trial interval that jittered betwixt 1000 and 1500 ms). Advantage consisted of a greenish smiley face up and the words 'You Win!'. Punishment consisted of a red sorry face and the words 'Y'all Lose'. Through trial and error, participants learned which image was associated with reward or with punishment (ie, expected reward and expected punishment trials; note that participants were not actually rewarded or punished, across receipt of this visual feedback). Reward and punishment associations would change after the participant got a random number (between five and ix) of sequent trials correct (ie, unexpected advantage and unexpected punishment trials; Cools et al, 2009). After a reversal/unexpected feedback trial, the aforementioned stimulus would also be highlighted on the very side by side trial. Following Cools et al, each bailiwick performed one practice block followed by iv boosted blocks, each consisting of 120 trials (Cools et al, 2009). The practice cake included 1 reversal, following which participants had to get 20 (not necessarily consecutive) trials correct earlier moving on to the experimental blocks, with this criterion imposed to ensure that participants were acclimated to the job (Cools et al, 2009; see Supplementary Methods for more details about the task).
We examined the FRN on these unexpected reversal trials that involve a change in reward vs punishment stimulus mapping that then result in participants getting unexpected feedback that they have made an error, and we compared the FRN for unexpected reward trials vs unexpected punishment trials. We examined behavioral learning from unexpected reward and unexpected punishment past examining reaction fourth dimension (RT) and accuracy on the trial immediately following unexpected feedback. Nosotros computed Advantage Sensitivity Differences Scores (for RT: unexpected punishment minus unexpected reward; for accuracy: unexpected reward minus unexpected punishment), with college reward sensitivity difference scores indicating faster and more than authentic learning after unexpected reward than afterwards unexpected penalty. In add-on, in social club to examine whether results were specific to the unexpected advantage and unexpected penalization trials, we also examined expected reward and expected punishment trials (ie, trials that did not involve a change in reward or punishment stimulus mappings).
Electrophysiological Recording and Analysis
Consistent with previous inquiry, we examined the FRN every bit the average voltage deflection between 225 and 325 ms post-result/feedback (eg, Bismark et al, 2013; electrophysiological recording details can be constitute in the Supplementary Methods). The FRNs at the frontocentral electrodes (F3, FZ, F4, FC3, FCZ, and FC4) were examined for each condition since previous research indicates that the FRN is maximal at frontocentral locations (Holroyd et al, 2003). The FRN was maximal at the FCZ electrode. The P3a was measured as the average voltage deflection between 350 and 500 ms mail-result/feedback at the FCZ and CZ electrodes (ie, where the P3a was maximal; Evans et al, 2013). The P3b was measured as the boilerplate voltage deflection between 500 and 600 ms post-outcome/feedback at the PZ electrode, where the P3b was maximal.
Process and Data Analyses
After completion of the questionnaire measures and the SIPS (Karcher et al, 2015b), eligible participants were invited to participate in the electric current study. Afterwards electrode placement while in a soundproof recording bedchamber, participants practiced and completed the RLT (as well every bit another, unrelated task non reported here), presented using Due east-Prime software (Psychology Software Tools, Inc., 2006).
Reaction times <200 ms and >10 000 ms were excluded from analyses. Cohen's d is included as a mensurate of effect size, except for F tests with numerator⩾2 that report eta-squared. Owing to excessive artifacts, EEG data from 2 participants (one PE and one control) had to be excluded from analyses.
The data were analyzed with mixed hierarchical linear modeling (HLM; Bates, 2010), which has several advantages over traditional repeated-measures analysis of variance for analyzing EEG information, particularly when sample sizes are modest (meet Luck, 2005; Page-Gould, in printing). The HLM approach does non assume the data run into the criterion of sphericity (ie, that the variances of the differences between all grouping combinations are equal), an assumption that is frequently violated in psychophysiological data (Jennings and Woods, 1976), and therefore corrections for this violation that can decrease statistical power (eg, Greenhouse–Geisser p-value adjustments) are non necessary. Further, the employ of an intercept for each electrode inside each subject also helps to reduce error variance estimates, which likewise helps to increase statistical power. Finally, since HLM does not use listwise deletion for missing information, this approach is more than robust to missing data, such as assuasive bad electrodes to exist rejected on an individual subject basis (Gratton, 2007). All ERP analyses examined amplitude using a 3 (group: PE, SocAnh, control) × 2 valence (reward, penalty) HLM with subject modeled as a random intercept.
RESULTS
Behavioral Task Performance
Reaction time
For trials immediately following unexpected feedback, in a trial type (unexpected reward, unexpected punishment) × group ANOVA, there was a master effect of trial type, F(one, 59)=5.33, p<0.05, d=0.60 (Table ane,Figure 1), with faster responses later on unexpected reward (M=867.02, SD=218.00) than unexpected punishment (M=912.51, SD=239.14). There was also a master result of group, F(2,59)=4.05, p<0.05, η 2 =0.120, with the PE group slower overall after unexpected feedback (M=1000.24, SD=215.09); vs control grouping (M=847.00, SD=227.02), t(38)=2.xiv, p<0.05, d=0.68; vs SocAnh (Thousand=832.79, SD=166.47), t(xl)=ii.79, p<0.01, d=0.86 (control vs SocAnh p=0.82, d=−0.07).
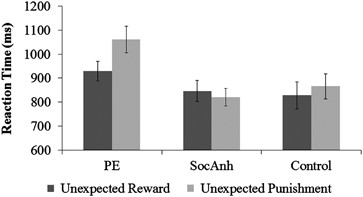
Meliorate learning afterwards unexpected reward than punishment on the Reversal Learning Task in the psychotic experiences group (PE). Reaction times are for the trial subsequently the unexpected reward and the unexpected punishment trials. Mistake bars reflect SE.
PowerPoint slide
Most chiefly, at that place was a predicted trial type 10 grouping interaction, F(two,59)=5.00, p<0.05, η 2 =0.144. Both control and SocAnh groups did not differ by unexpected feedback type; command p=0.35, d=0.16; SocAnh p<0.50, d=−0.14. In dissimilarity, the PE group was faster for unexpected reward than for unexpected penalty, t(eighteen)=4.93, p<0.001, d=1.27. Farther, the difference in RT between unexpected reward and unexpected punishment (ie, the Reward Sensitivity Divergence Score) was larger in the PE group (M=127.19, SD=120.96) compared with the SocAnh group (M=−26.56, SD=183.25), t(twoscore)=3.29, p<0.005, d=1.04; the departure was marginally larger in the PE grouping compared with the control grouping (M=37.76, SD=176.69), t(38)=1.98, p=0.06, d=0.63 (control and SocAnh groups did not differ, p=0.26, d=0.37). Hence, as expected, PE appeared to be associated behaviorally with increased sensitivity to advantage relative to penalty. In a comparable assay of accuracy, there were no pregnant main effects or interaction (Fs<1).
FRN Amplitude
In the HLM on FRN amplitude, the effects of valence (p=0.13, d=−0.forty) and group (p=0.85, d=0.05) were not significant. However, every bit expected, at that place was a meaning Valence × Group interaction, t(675)=3.51, p<0.0005, d=0.91 (Tabular array 2 and Figure 2). Both command and SocAnh groups exhibited the typical pattern of larger (ie, more than negative) FRN to unexpected penalisation feedback than to unexpected reward feedback; control t(213.84)=3.59, p<0.001, d=1.65; SocAnh t(235.95)=4.37, p<0.001, d=i.91. In contrast, the PE group generally showed like FRNs to unexpected punishment and unexpected reward, t(213.99)=−1.15, p=0.25, d=−0.53; if annihilation, unexpected advantage elicited numerically larger FRNs than unexpected punishment in the PE group (Figure 2d).
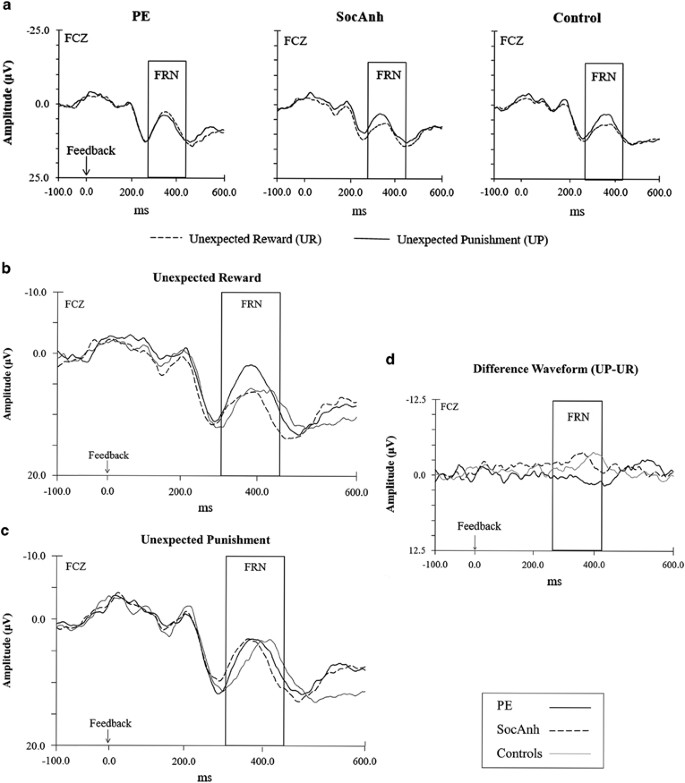
The psychotic experiences (PE) group showed like FRNs to punishment and reward, with a numerically larger feedback-related negativity (FRN) to advantage than punishment (225–325 ms post-stimulus) elicited at the FCZ electrode for each of the groups. Note that post-obit common upshot-related potential (ERP) enquiry convention and because the FRN is a negative deflection, negative values are plotted upwards. (a) Grand boilerplate waveforms comparing the unexpected advantage (UR) and unexpected penalization (Upwardly) conditions for each of the groups. (b) One thousand average waveform for the unexpected reward (UR) condition. (c) 1000 average waveform for the unexpected punishment (UP). (d) Grand boilerplate departure waveform for Reward Sensitivity Differences Score (UP FRN−UR FRN).
PowerPoint slide
Additional comparisons showed that the magnitude of the FRN to unexpected punishment did not differ across the groups, F(two,59)=0.29, p=0.75, η 2=0.00. In dissimilarity, the FRN elicited past unexpected reward feedback differed significantly across the groups, F(2,59)=5.93, p<0.005, η 2=0.03, with the PE grouping showing a larger FRN in comparison with the other ii groups (Figure 2b).
Boosted Analyses
We performed several additional analyses to examine whether results for the PE group were specific to unexpected feedback trials and whether they were specific to the FRN (total results reported in the Supplementary Results Section). First, we examined behavioral functioning and the FRN after correct expected feedback trials. Here there were no significant behavioral or FRN effects for group. Hence, significant FRN grouping differences for PE vs the other two groups were specific to unexpected feedback trials.
Next nosotros examined whether the pregnant group differences for the FRN reported previously could accept reflected differences in the P3a (ie, whether what appears to be a larger FRN is actually due to a smaller P3a in frontocentral electrodes). Here, just as for the FRN, in that location was a significant Valence × Group interaction, t(180.77)=−two.91, p<0.005, d=−0.76 (Table iii). Both command and SocAnh groups exhibited not-significantly larger P3a for unexpected punishment than unexpected reward trials; controls p=0.nineteen, d=−0.61; SocAnh p=0.65, d=−0.20 (with these groups non differing from each other, p=0.48, d=−0.22). In contrast, the PE group showed a significantly larger P3a for unexpected advantage trials than unexpected punishment trials, t(56.51)=ii.52, p<0.05, d=i.16, and the P3a to unexpected reward feedback was significantly larger in the PE group compared with both the control, t(115.54)=−2.79, p<0.01, d=−0.91 and SocAnh groups, t(121.52)=−2.32, p<0.05, d=−0.73. Hence, just every bit for the FRN, the PE group exhibited a larger P3a for unexpected reward than unexpected penalisation, and therefore it is articulate that the association betwixt the FRN and PE cannot exist accounted for by a reduction in the P3a.
DISCUSSION
Very few studies have linked either PE or anhedonia in at-risk populations to a neural response evoked by a reward and punishment-based learning task. The current enquiry provides novel evidence that people with PE showroom behavioral and neural deficits consistent with aberrant reward and penalisation-based learning. In contrast to psychosis take a chance, the electric current results for SocAnh were quite different from PE. Hence, this suggests that in the current study reward and penalty-based learning dysfunction was specific to PE and therefore cannot be hands attributable to a general adventure for schizophrenia-spectrum disorders. These results could help us better understand the nature of psychosis risk and also might prove useful in better identifying people at risk for psychotic disorders.
In terms of their behavior, individuals in the PE grouping exhibited greater sensitivity to learning from unexpected advantage than from unexpected punishment, as reaction times on trials afterward unexpected reward were significantly faster than after unexpected punishment. Previous research using this version of the RLT has found consequent evidence that increased striatal dopamine is associated with increased sensitivity to learning from unexpected reward (van der Schaaf et al, 2014). Similarly, other behavioral research too has institute that increased dopamine in the striatum is related to increased sensitivity to learning from rewards (Frank et al, 2004). Hence, the current bear witness of PE being associated with greater sensitivity to learning from unexpected reward than from unexpected punishment is consistent with increased dopamine in the striatum in people with psychosis risk (Karcher et al, 2015b).
In addition to PE being associated with increased behavioral sensitivity to reward, while the command and SocAnh groups showed a larger FRN to penalty than reward, the PE group showed similar FRNs to penalty and reward, with a numerically larger FRN to advantage than punishment. Moreover, whereas the groups did not differ in their FRN responses to unexpected punishment, the FRN to unexpected reward was larger in the PE group than in the other two groups. A relatively increased neural response for unexpected advantage in psychosis take a chance is consistent with previous evidence on striatal dopamine and the FRN. Dopamine agonists have been found to increase the FRN for unexpected reward (Santesso et al, 2009). The FRN has been plant to increase for salient stimuli (Pfabigan et al, 2015), and there is evidence that increased striatal dopamine enhances processing of rewarding data (Collins and Frank, 2014). There is also evidence that the FRN to advantage increases with increased reward sensitivity (Smillie et al, 2011). Hence, the current FRN results in the PE group are consistent with an increase of striatal dopamine in psychosis take a chance. Moreover, in additional analyses, results for the P3a were similar to the results for the FRN, with larger neural responses to reward than to punishment in the PE group. Therefore, in the electric current study multiple behavioral and neural indicators were consistent with the possibility that psychosis take a chance is associated with increased striatal dopamine. The current results propose that behavioral and electrophysiological measures have the potential to successfully identify people at hazard for psychosis past providing prove of dopamine dysregulation (Wagshal et al, 2012; Weickert et al, 2010). Furthermore, it should be noted that the results of the current study differ from the results of a previous study examining reversal learning (von Borries et al, 2013), which found that the FRN varied every bit a function of valence, but not expectancy (ie, in that study there was not a meaning difference in the FRN for expected vs unexpected trials). Compared with our study, that previous report had smaller runs of consecutive expected trials, which may have reduced the deviation between expected and unexpected trials.
1 consequence for future psychosis risk enquiry is to continue to examine the nature of striatal dysfunction observed in the current study. For instance, information technology is possible that the FRN is the effect of 2 functionally and empirically singled-out neural responses that overlap in time, one that is more than reflective of feedback salience, reflecting the N2, and a second that is more cogitating of positive valence, labeled positivity to rewards (RewP; Holroyd and Coles, 2002; Proudfit, 2015). The electric current ERP results for the PE group presumably reflect increased salience to advantage feedback and an increased N2, rather than an altered RewP. Future research could attempt to separately appraise these two FRN-related components, maybe using principal components assay, and examine which 1 is associated with psychosis risk. Another estimation of the altered FRN in the PE group is a lack of sensitivity to upshot valence, given that the PE group did not exhibit a deviation in the FRN for reward vs punishment trials. Although the FRN results could propose a lack of outcome sensitivity in the PE grouping, there was other evidence (behavioral and P3a) that this group was more sensitive to advantage vs penalty result information than the other groups, which maybe could also explain the FRN results in the PE group. Hence, one effect for future research would be to further examine whether the FRN results in the PE group reflect a lack of sensitivity to outcome valence or an increased sensitivity to reward vs penalization information. In addition, time to come enquiry could examine performance on the RLT in people with psychosis take chances using fMRI to examine functioning of the striatum and other potentially relevant brain regions (eg, ACC) during this task. As well, one limitation of the report is that we did not appraise medication usage. However, in a recent study utilizing the same recruitment method, none of the individuals in the PE had a history of antipsychotic medication usage. Therefore, information technology seems reasonable to expect that none, or at the most perhaps a very modest number, of the people in the PE group in the sample for the current manuscript had ever previously taken antipsychotic medication.
In contrast to the PE group, the social anhedonia grouping did not differ from the command grouping in their behavioral performance or in their FRN for unexpected trials on the RLT, suggesting that some aspects of reward and punishment-based learning may to some extent be intact in people with extremely elevated social anhedonia who are at increased take a chance for schizophrenia-spectrum disorders (Karcher et al, 2015b; Padrao et al, 2013). Future inquiry should keep to examine whether some particular aspect of reward and penalisation-based learning, perhaps reward anticipation (Dowd and Barch, 2012; Juckel et al, 2006), is associated with anhedonia in at hazard groups.
Funding and disclosure
Dr Bartholow has received research funding from the National Institutes of Health (NIH) and has reviewed grant proposals for the NIH and other agencies; has served as Associate Editor for bookish journals; and has given bookish lectures at numerous universities and medical centers. Dr Martin has consulted for Evidera and provided clinical care at McLean Infirmary/Harvard Medical School. She received support through a Hellman Fellowship given by the Academy of California and performed grant reviews for NSF. Dr Kerns has received grant funding from National Institutes of Mental Wellness (NIMH) and has reviewed grant proposals for NIH. The authors declare no conflict of interest.
References
-
Baker TE, Holroyd CB (2011). Dissociated roles of the anterior cingulate cortex in advantage and conflict processing as revealed by the feedback mistake-related negativity and N200. Biol Psychol 87: 25–34.
-
Bates DM (2010). lme4: Mixed-effects modeling with R. Retrieved from http://lme4.r-forge.r-projection.org/lMMwR/lrgprt.pdf.
-
Becker MP, Nitsch AM, Miltner WH, Straube T (2014). A single-trial estimation of the feedback-related negativity and its relation to BOLD responses in a time-estimation task. J Neurosci 34: 3005–3012.
-
Bismark AW, Hajcak One thousand, Whitworth NM, Allen JJ (2013). The role of outcome expectations in the generation of the feedback‐related negativity. Psychophysiology 50: 125–133.
-
Carlson JM, Foti D, Mujica-Parodi LR, Harmon-Jones E, Hajcak Grand (2011). Ventral striatal and medial prefrontal Bold activation is correlated with advantage-related electrocortical activity: a combined ERP and fMRI study. Neuroimage 57: 1608–1616.
-
Chapman LJ, Chapman JP, Kwapil TR, Eckblad M, Zinser MC (1994). Putatively psychosis-prone subjects 10 years later. J Abnorm Psychol 103: 171–183.
-
Chapman LJ, Chapman JP, Raulin ML (1978). Trunk-image aberration in schizophrenia. J Abnorm Psychol 87: 399–407.
-
Collins AG, Frank MJ (2014). Opponent actor learning (OpAL): modeling interactive effects of striatal dopamine on reinforcement learning and choice incentive. Psychol Rev 121: 337–366.
-
Cools R, Frank MJ, Gibbs SE, Miyakawa A, Jagust W, D'Esposito K (2009). Striatal dopamine predicts outcome-specific reversal learning and its sensitivity to dopaminergic drug administration. J Neurosci 29: 1538–1543.
-
Debbané M, Eliez Due south, Badoud D, Conus P, Flückiger R, Schultze-Lutter F (2015). Developing psychosis and its risk states through the lens of schizotypy. Schizophr Bull 41: S396–S407.
-
Demjaha A, Murray RM, McGuire PK, Kapur S, Howes OD (2012). Dopamine synthesis capacity in patients with handling-resistant schizophrenia. Am J Psychiatry 169: 1203–1210.
-
Dowd EC, Barch DM (2012). Pavlovian advantage prediction and receipt in schizophrenia: relationship to anhedonia. PLoS ONE 7: e35622.
-
Eckblad M, Chapman LJ (1983). Magical ideation equally an indicator of schizotypy. J Consult Clin Psychol 51: 215–225.
-
Eckblad Yard, Chapman LJ, Chapman JP, Mishlove M (1982). The revised social anhedonia scale. Unpublished test, Academy of Wisconsin, Madison, WI.
-
Evans DE, Maxfield ND, Van Rensburg KJ, Oliver JA, Jentink KG, Drobes DJ (2013). Nicotine deprivation influences P300 markers of cognitive command. Neuropsychopharmacology 38: 2525–2531.
-
Frank MJ, Seeberger LC, O'Reilly RC (2004). Past carrot or past stick: cognitive reinforcement learning in parkinsonism. Scientific discipline 306: 1940–1943.
-
Gratton Grand (2007) Biosignal processing. In: Cacioppo J, Tassinary 50, Berntson G (eds). Handbook of Psychophysiology. Cambridge Academy Press: New York. pp 900–923.
-
Holroyd CB, Coles MG (2002). The neural ground of human error processing: reinforcement learning, dopamine, and the error-related negativity. Psychol Rev 109: 679–709.
-
Holroyd CB, Nieuwenhuis Southward, Yeung N, Cohen JD (2003). Errors in advantage prediction are reflected in the event-related brain potential. Neuroreport 14: 2481–2484.
-
Howes O, Kapur S (2009). The dopamine hypothesis of schizophrenia: Version Iii—The final common pathway. Schizophr Bull 35: 549–562.
-
Jennings JR, Wood CC (1976). The e-adjustment procedure for repeated-measures analyses of variance. Psychophysiology 76: 277–278.
-
Juckel G, Schlagenhauf F, Koslowski M, Wustenberg T, Villringer A, Knutson B et al (2006). Dysfunction of ventral striatal advantage prediction in schizophrenia. Neuroimage 29: 409–416.
-
Karcher NR, Cicero D, Kerns JG (2015a). An experimental test of the abnormal salience hypothesis using a salience manipulation and a behavioral magical thinking task. J Exp Psychopathol 6: 297–312.
-
Karcher NR, Martin EA, Kerns JG (2015b). Examining associations between psychosis risk, social anhedonia, and performance of striatum-related behavioral tasks. J Abnorm Psychol 124: 507–518.
-
Kaymaz Northward, Drukker Yard, Lieb R, Wittchen HU, Werbeloff Due north, Weiser Yard et al (2012). Practise subthreshold psychotic experiences predict clinical outcomes in unselected non-help-seeking population-based samples? A systematic review and meta-analysis, enriched with new results. Psychol Med 42: 2239–2253.
-
Luck SJ (2005) An Introduction to the Event-Related Potential Technique. MIT Press: Cambridge.
-
Miller TJ, McGlashan TH, Rosen JL, Cadenhead Thou, Cannon T, Ventura J et al (2003). Prodromal assessment with the structured interview for prodromal syndromes and the scale of prodromal symptoms: predictive validity, interrater reliability, and training to reliability. Schizophr Balderdash 29: 703–715.
-
Nieuwenhuis Due south, Slagter HA, von Geusau NJ, Heslenfeld DJ, Holroyd CB (2005). Knowing good from bad: differential activation of human cortical areas by positive and negative outcomes. Eur J Neurosci 21: 3161–3168.
-
Padrao G, Mallorqui A, Cucurell D, Marco-Pallares J, Rodriguez-Fornells A (2013). Neurophysiological differences in reward processing in anhedonics. Cogn Affect Behav Neurosci xiii: 102–115.
-
Folio-Gould E Multilevel modeling . Cacioppo JT, Tassinary Fifty, Berntson G (eds). The Handbook of Psychophysiology (in press)4th edn. Cambridge University Press: New York.
-
Pfabigan DM, Seidel EM, Paul M, Grahl A, Sailer U, Lanzenberger R et al (2015). Context-sensitivity of the feedback-related negativity for zilch-value feedback outcomes. Biol Psychol 104: 184–192.
-
Polich J (2007). Updating P300: An integrative theory of P3a and P3b. Clin Neurophysiol 118: 2128–2148.
-
Proudfit GH (2015). The reward positivity: From basic research on reward to a biomarker for low. Psychophysiology 52: 449–459.
-
Psychology Software Tools, Inc. (2006). East-prime v. 2.0. Pittsburg, PA.
-
Santesso DL, Evins AE, Frank MJ, Schetter EC, Bogdan R, Pizzagalli DA (2009). Single dose of a dopamine agonist impairs reinforcement learning in humans: prove from event-related potentials and computational modeling of striatal-cortical office. Hum Encephalon Mapp 30: 1963–1976.
-
Smillie LD, Cooper AJ, Pickering AD (2011). Individual differences in advantage-prediction-error: extraversion and feedback-related negativity. Soc Cogn Affect Neurosci vi: 646–652.
-
van der Schaaf ME, van Schouwenburg MR, Geurts DE, Schellekens AF, Buitelaar JK, Verkes RJ et al (2014). Establishing the dopamine dependency of human striatal signals during advantage and punishment reversal learning. Cereb Cortex 24: 633–642.
-
von Borries AK, Verkes RJ, Bulten BH, Cools R, de Bruijn ER (2013). Feedback-related negativity codes outcome valence, merely not outcome expectancy, during reversal learning. Cogn Affect Behav Neurosci 13: 737–746.
-
Wagshal D, Knowlton B, Cohen J, Poldrack R, Bookheimer Due south, Bilder R et al (2012). Deficits in probabilistic classification learning and liability for schizophrenia. Psychiatry Res 200: 167–172.
-
Walsh MM, Anderson JR (2012). Learning from feel: event-related potential correlates of advantage processing, neural adaptation, and behavioral choice. Neurosci Biobehav Rev 36: 1870–1884.
-
Weickert TW, Goldberg TE, Egan MF, Apud JA, Meeter Thou, Myers CE et al (2010). Relative adventure of probabilistic category learning deficits in patients with schizophrenia and their siblings. Biol Psychiatry 67: 948–955.
Author information
Affiliations
Corresponding writer
Additional information
Supplementary Information accompanies the paper on the Neuropsychopharmacology website
Supplementary information
PowerPoint slides
Rights and permissions
Virtually this article
Cite this article
Karcher, North., Bartholow, B., Martin, E. et al. Associations between Electrophysiological Evidence of Reward and Punishment-Based Learning and Psychotic Experiences and Social Anhedonia in At-Chance Groups. Neuropsychopharmacol 42, 925–932 (2017). https://doi.org/10.1038/npp.2016.192
-
Received:
-
Revised:
-
Accustomed:
-
Published:
-
Effect Date:
-
DOI : https://doi.org/ten.1038/npp.2016.192
Further reading
Source: https://www.nature.com/articles/npp2016192
0 Response to "Reversal Learning Task Eeg Study(Karcher Et Al 2017 Review"
Post a Comment